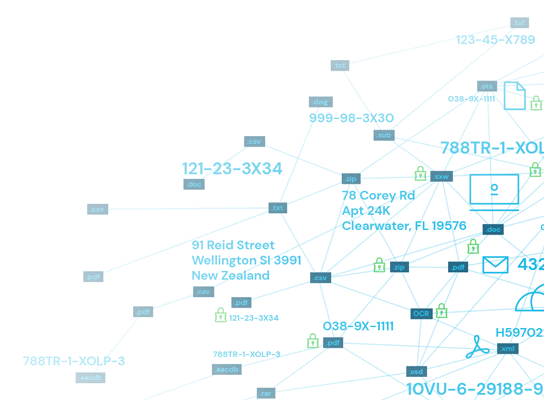
Data Classification Guide
Data Classification Guide
A Critical First-Step in the Battle to Keep Sensitive Data Private, Secure, and in Compliance
Today’s organizations create, store, and manage more information than ever before, including sensitive data, such as spreadsheets containing employees’ Social Security numbers. Keeping this abundance of data private, secure, and in compliance requires a higher level of data management and control than ever before. This requires organizations to install a range of tools and practices. One of the leading privacy tools and practices is data classification.
What is data classification?
Data classification is the process of separating and organizing data into relevant groups (“classes”) based on their shared characteristics, such as their level of sensitivity, the risks they present, and the compliance regulations that protect them. To protect sensitive data, it must be located, classified according to its level of sensitivity, and accurately tagged. Then, enterprises must handle each group of data in ways that ensure only authorized people can gain access, both internally and externally, and that the data is always handled in full compliance with all relevant regulations.
When done correctly, data classification makes using and protecting data easier and more efficient. However, this process is often overlooked, especially when organizations don’t understand its full purpose, scope, and capabilities.
This guide provides a comprehensive overview of data classification to help organizations understand how it works and where it fits within an enterprise data security and privacy program.
Data classification basics
This section provides an overview of key concepts related to data classification and answers basic questions about the role of data classification within an organization’s comprehensive data privacy, security, and compliance programs.
Why classify your data?
Data security and privacy suffer if organizations don’t know their data, including where it lives and how it needs to be protected. To “know your data” means having understanding where all “sensitive” data is located across an enterprise. According to Forrester, data privacy professionals, such as Data Privacy Officers (DPO), cannot effectively protect customer, employee, and corporate information if they don’t know the following:
- What data exists across their enterprise
- Where it resides exactly
- Its value and risk to the organization
- Compliance regulations governing the data
- Who is allowed to access and use the data
Data classification delivers this insight by providing a consistent process that identifies and tags all sensitive information wherever it resides across an enterprise — such as in networks, sharing platforms, endpoints, and cloud files. It works by enabling the creation of attributes for data that prescribe how to handle and secure each group according to corporate and regulatory requirements. Because the data is easy to find, organizations can apply protections that lower data exposure risks, reduce the data footprint, eliminate data protection redundancies, and focus security resources on the right actions. In this way, classification both streamlines and strengthens organizations’ data privacy and security protection programs.
Benefits of data classification
Only 54% of companies know where their sensitive data is stored. This dark data is a serious problem in the fight to keep sensitive data secure, private, and in compliance. By launching comprehensive, well-planned data classification programs, organizations gain a wide range of benefits.
Improve data security
Data classification enables organizations to safeguard sensitive corporate and customer data by answering the following critical questions:
- What sensitive data do we have (IP, PHI, PII, credit card, etc.)?
- Where does this sensitive data reside?
- Who can access, modify, and delete it?
- How will it affect our business if the data is leaked, destroyed, or improperly altered?
Knowing the answers to these questions delivers several benefits, including:
- Decrease the sensitive data footprints, thereby, making data security more effective.
- Reduces access to sensitive data to only approved users.
- Understand the criticality of different types of data, so they can be better protected.
- Install the right data protection technologies, such as encryption, data loss prevention (DLP), and identity loss and protection (ILP).
- Optimize costs without wasting resources on non- or less-critical data.
Support regulatory compliance
Data classification helps determine where regulated data is located across the enterprise, ensures that appropriate security controls are in place, and that the data is traceable and searchable, as required by compliance regulations. This delivers these advantages:
- Ensures that sensitive data is handled appropriately for different regulations, such as medical, credit card, and personally identifiable information (PII).
- Aids in the ability to maintain day-to-day compliance with all relevant rules, regulations, and privacy laws.
- Supports rapid retrieval of specific information within a set timeframe, which helps meet newer compliance rules.
- Demonstrates organizations’ expertise and support of programs that ensure data privacy compliance.
- Improves the opportunity to pass compliance audits.
Boost business operation efficiency and lowers business risks
From the time information is created until it is destroyed, data classification can help organizations ensure they are effectively protecting, storing, and managing their data. This delivers the following benefits:
- Provides better insight into and control over the data that organizations hold and share.
- Enables more efficient access to and use of protected data across the organization.
- Facilitates risk management by helping organizations assess the value of their data and the impact of it being lost, stolen, misused, or compromised.
- Contributes valuable capabilities for record retention and legal discovery.
Challenges of data classification
Almost every organization houses some types of sensitive data — often much more than they realize. However, it’s unlikely they understand exactly where that data lives throughout their infrastructure and the many ways it could be accessed or compromised. For this reason and others, establishing effective data classification programs within organizations faces a wide range of challenges.
Data classification can be expensive and cumbersome
Few organizations are equipped to handle data classification by traditional (manual) methods. This creates several challenges, including:
- Sensitive data has the potential to become lost in data silos where it is undiscoverable and unprotected.
- Mishandling of sensitive information can result in embarrassment for clients and loss of future revenue.
- Organizations can be fined and penalized for mishandling regulated data.
- Breached client information can spawn lawsuits, tarnish an organization’s reputation, and lower goodwill.
Lack understanding of data classification best practices
Poor execution of data classification can result in a cascading series of data security and privacy failures, resulting in these challenges:
- Leadership has an “it won’t happen to us” mindset.
- Data and privacy concerns get put in line behind other pressing priorities, such as sales, marketing, expansion, and product expenses.
- Companies don’t know how to locate or identify their data.
- Organizations are out of sync with ever-evolving compliance regulations.
- Companies make data classification overly complex, thereby, failing to produce practical results.
Lack of enforcement of data privacy policies
Many organizations have data classification policies that are theoretical rather than operational. In other words, the corporate policy is not enforced, or it’s left to business users and data owners to implement.
The challenge stems from overlooking answering critical questions such as:
- Are inappropriate data privacy discussions happening at the top levels in an organization?
- Who is ultimately responsible for data privacy, and do they have the powers to implement and control solutions?
- Is sensitive and confidential information being shared with other entities?
- Are privacy and compliance policies being circumvented, either deliberately or inadvertently?
Where does data classification fit in the data lifecycle?
The data lifecycle provides an ideal structure for controlling the flow of data across an enterprise. Organizations need to account for data security, privacy, and compliance at every step. Data classification can help because it can be enacted at every state — from creation to deletion.
The data lifecycle includes these six stages:
- Creation — Sensitive data is generated in multiple formats, including emails, Excel documents, Word documents, Google documents, social media, and websites.
- Role-based use — Role-based security controls are applied to all sensitive data via tagging based on internal security policies and compliance rules.
- Storage — After every use, data is stored with access controls and encryption.
- Sharing — Data is constantly being shared among employees, customers, and partners from different devices and platforms.
- Archive — Most data is eventually archived within an organization’s storage systems.
- Permanently destroy — Significant amounts of data need to be destroyed to reduce the storage burden and improve overall data security.
Data should be classified as soon as it’s created. As data moves through the stages of the data lifecycle, classification should be continually evaluated and updated.
Data classification and data discovery
Along with data classification, comprehensive data privacy and security programs include a wide variety of tasks. Among these tasks is data discovery, another critical step in data privacy. This is the process of collecting data from databases and silos and consolidating it into a single source that can be easily and instantly accessed. Data classification and data discovery go hand-in-hand.
Together, data discovery and classification make data more secure by providing the critical first step in a comprehensive data privacy and security program. In fact, data discovery and classification is the first phase of Forrester’s Data Security and Control Framework, which breaks down data protection into three areas: 1) defining data, 2) dissecting and analyzing data, and 3) defending data.
Going even further, the data classification and discovery process can be made more efficient through automation. By automating the classification process, many of the inefficiencies of manual classification can be addressed, including accuracy, subjectivity, inconsistency, and more. Data classification software tools offer exceptional benefits for an organization thanks to the way these solutions directly address these concerns.
How data classification works
This section drills down into the nuts and bolts of data classification — providing critical insight on everything from the types of data that need to be classified to compliance regulations governing data privacy and security to the roles involved in data classification within organizations.
3 types of data classification systems
There are three options for creating data classification programs:
- Manual — Traditional data classification methods require human intervention and enforcement.
- Automated — Technology-driven solutions eliminate the risks of human intervention, including excessive time and errors, while adding persistence (around-the-clock classification of all data).
- Hybrid — Human intervention provides context for data classification, while tools enable efficiency and policy enforcement.
Assessing data classification levels
Organizations typically design their own data classification models and categories. For example, U.S. government agencies often define three data types: public, secret, and top secret. Organizations in the private sector usually start by classifying data in these three categories: restricted, private, or public.
Too often organizations fall into a data privacy pitfall when they use overly complex and haphazard legacy classification processes. But data classification does not have to be complicated. In fact, a best practice is to create an initial data classification model with three or four data classification levels. Then later add more granular levels based on an organization’s specific data, compliance requirements, and other business needs.
Determining an organization’s data classification levels begins with determining sensitivity of data across the enterprise. As the potential impact moves from low to high, the sensitivity increases and, therefore, the classification level of data should become higher and more restrictive. The National Institute of Standards and Technology (NIST) provides a guide for this process: the Federal Information Processing Standards (FIPS) 199 publication. It provides a framework for determining the sensitivity of information according to three key criteria.
Confidentiality — Preserves authorized restrictions on information access and disclosure, including the means for protecting personal privacy and proprietary information. The unauthorized disclosure of information could be expected to have a limited (low), serious (moderate), or severe/catastrophic (high) adverse effect on organizational operations, organizational assets, or individuals.
Integrity — Guards against improper information modification or destruction, and includes ensuring information nonrepudiation and authenticity. The unauthorized modification or destruction of information could be expected to have a limited (low), serious (moderate), or severe/catastrophic (high) adverse effect on organizational operations, organizational assets, or individuals.
Availability — Ensures timely and reliable access to and use of information. The disruption of access to or use of information or an information system could be expected to have a limited (low), serious (moderate), or severe/catastrophic (high) adverse effect on organizational operations, organizational assets, or individuals.
Another way to assess the value and risk of sensitive across an organization is to ask these key questions:
- Criticality — How important is the data for everyday operations and business continuity?
- Availability — Is timely and reliable access to the data important for the business?
- Sensitivity — What is the potential impact on the business if the data is compromised?
- Integrity — How important is it to ensure that the data is not tampered with during storage or while in transit?
- Retainability — How long must the data be retained according to regulatory requirements or industry standards?
Types of data to be classified
Almost every organization houses some type of sensitive data — often much more than they realize. Each must understand the specific types of sensitive data within their enterprises and execute data classification in ways that support optimized data privacy, security, and compliance.
Data that needs to be classified is often called “sensitive data.” This means that if it is exposed inside or outside of the organization it presents risks to individuals’ privacy and security, or that it risks falling out of compliance with leading data protection regulations.
It’s estimated that the identity of 87% of Americans can be determined using a combination of the person’s gender, date of birth, and ZIP code. When taken separately, these details might not seem sensitive. However, a breach of those three elements would likely also compromise the individual’s name, home address, Social Security number, and other personal data. As a result, those elements should be considered sensitive.
Overall, data housed within today’s organizations can be categorized into two broad categories: regulated and unregulated data (by compliance agencies). General types of information fall under each main category.
Regulated information
Data that is regulated by compliance organizations is always sensitive, though to varying degrees, and should always be classified. This includes:
Personally Identifiable Information (PII) — Data that could be used to identify, contact, or locate a specific individual or distinguish one person from another: this information includes social security numbers, drivers’ license numbers, addresses, and phone numbers.
Personal Health Information (PHI) — A person’s health and medical information, such as insurance, tests, and health status.
Financial Information — A person’s financial information, such as credit card numbers, bank account information, and passwords.
Unregulated information
In many cases, unregulated data is highly sensitive and critical to protect. This includes:
Authentication Information — Data used to prove the identity of an individual, system, or service, such as passwords, shared secrets, encryption keys, and hash tables.
Corporate Intellectual Property — This includes organizations’ unique information, such as intellectual property, business plans, trade secrets, and financial records.
Government Information — Any information that is classified as secret or top-secret, restricted, or can be considered a breach of confidentiality if exposed.
Compliance regulations overview
Most sensitive data in today’s enterprises is regulated by several compliance agencies, including local, state, and national regulations. Among the many compliance rules that cover data privacy, there are four main ones to which today’s organizations must adhere.
Health Insurance Portability and Accountability Act (HIPAA)
This regulation protects individuals’ protected health information (PHI). HIPAA has up to 18 identifiers of sensitive data that must be protected, including medical record numbers, health plan and health insurance beneficiary numbers, and biometric identifiers, such as fingerprints, voiceprints, and full-face photos. The HIPAA Privacy Rule requires organizations to ensure the integrity of electronic personal health information (ePHI).
HIPAA classification guidelines require organizations to group data according to its level of sensitivity, such as:
Restricted/confidential data — Data whose unauthorized disclosure, alteration, or destruction could cause significant damage. This data requires the highest level of security and controlled access in accordance with the principle of the least privilege.
Internal data — Data whose unauthorized disclosure, alteration, or destruction could cause low or moderate damage. This data is not for release to the public, and requires reasonable security controls.
Public data — Data that doesn’t need protection against unauthorized access, but does need protection against unauthorized modification or destruction.
Payment Card Industry Data Security Standard (PCI-DSS)
This regulation protects an individual’s payment card information, including credit card numbers, expiration dates, CVV codes, pins, and more. The PCI-DSS regulation has one identifier of sensitive data that must be protected: cardholder data.
Data classification is requested in terms of regular risk assessment and security categorization processes. Cardholder data elements should be classified according to their type, storage permissions, and required levels of protection to ensure that security controls apply to all sensitive data, as well as confirm that all instances of cardholder data are documented and that no cardholder data exists outside of the defined cardholder environment.
General Data Protection Regulation (GDPR)
This regulation protects the PII of European Union residents. The GDPR defines personal data as any information that can identify a natural person, directly or indirectly, such as:
- Names
- Identification numbers
- Location data
- Online identifiers
- One or more factors specific to the physical, physiological, genetic, mental, economic, cultural, or social identity of the person
To comply with the GDPR, organizations must classify data within a data inventory structure, including the following:
- Type of data (financial information, health data, etc.)
- Basis for data protection (personal or sensitive information)
- Categories of the individuals involved (customers, patients, etc.)
- Categories of recipients (especially international third-party vendors)
California Consumer Privacy Act (CCPA)
This regulation, taking effect on July 1, 2023, brings the key data privacy concepts of Europe’s GDPR onto American shores — specifically to California residents. It requires businesses that interact with California residents to adhere to a new set of obligations around consumer rights related to personal data that is collected, processed, or sold by companies that are covered by the law. The obligations include:
- Giving citizens the right to request information about what types of data a company has collected, the purpose of collecting it, and the names of companies to whom the data was sold.
- The right to opt-out of data collection or sale.
- The right to request deletion of personal data.
Three components of CPRA all companies will need to be aware of are:
- The new category of special personal information requiring protection that includes names, Social Security numbers, email addresses, and birthdays.
- The requirement to proactively introduce security measures to protect personal information.
- The increased regulation of service providers and contractors who have access to personal information held by a business.
Fulfilling the requirements of these four standard data privacy compliance regulations is nearly impossible without an intelligent data classification policy.
The Gramm-Leach-Bliley Act (GLBA)
Enacted in 1999, the Gramm-Leach-Bliley Act requires financial institutions to explain to their customers how information gathered by the institution is shared. The GLBA also sets forth requirements for securing sensitive data. There are three primary ways in which GLBA policies affect consumers:
- Financial institutions are required to secure confidential customer information, protect against threats to security and integrity, and prevent unauthorized access of customer information.
- Financial institutions must be able to explain how personal information is used and shared by the organization while also giving consumers the option to not have certain information shared if they so choose.
- Financial institutions must be able to explain to customers how their information will be secured and kept confidential.
This law applies to many types of institutions. While banks, credit unions, and savings and loan companies are clear examples of financial institutions covered by GLBA, additional industries covered include securities firms, car dealers, and retailers who collect and share personal information and provide credit to consumers.
Guidelines for data classification
There is no one-size-fits-all approach to creating a comprehensive and intelligent data classification program. However, the process can be broken down into seven key steps, all of which can be tailor to meet each organization’s unique needs.
Conduct a sensitive data risk assessment
Gain a comprehensive understanding of the organization’s corporate, regulatory, and contractual privacy and confidentiality requirements. Define data classification objectives with all stakeholders, including:
- Privacy leaders
- Security leaders
- Compliance leaders
- Legal leaders
Develop a formalized classification policy
An organization’s classification policy overviews the who, what, where, when, why, and how, so that everyone understands the role that data classification plays across the enterprise. Points to cover in the policy include:
Objectives — Overview the reasons why data classification has been put into place and the goals the company expects to achieve.
Workflows — Explain how the classification process will be organized and how it will impact employees who use different categories of sensitive data.
Schema — Describe the data categories that will be used to classify the organization’s data.
Data owners — Outline the roles and responsibilities of everyone involved in managing data classification, and how they classify sensitive data and grant access.
Categorize the types of data
Each business will define sensitive data differently. Plus, state and federal regulations define sensitivity differently. Determine what types of sensitive data exist within the organization. To complete this task, ask the following questions:
- What customer and partner data does the organization collect?
- How is the data used?
- What proprietary data is created?
- What are the confidentiality and risk levels of the data collected across the enterprise?
- Which privacy regulations rules apply to the data?
Discover the location of all data
Catalog all of the places that data is stored across the enterprise, including within:
- Network
- Endpoints
- Devices
- Cloud
Identify and classify data
After locating data using data discovery methods, identify and classify it so that it’s appropriately protected. Give each sensitive data asset a label to improve data classification policy enforcement. Labeling can be automated in accordance with your data classification scheme or done manually by data owners. Intelligent automated classification systems deliver these advantages:
- Automatically determination of appropriate classifications for all data across the enterprise based on organization-approved methodologies.
- Tag the data with the appropriate classification level label.
- Persistently ensure that all data is classified and updated as it moves through the data lifecycle.
Enable effective data security controls
Establish baseline cybersecurity measures and define policy-based controls for each data classification label to ensure the appropriate security solutions are in place. By understanding where data resides and the organizational value of the data, you can implement appropriate security controls based on associated risks. Also, classification metadata can be used by DLP, ILP, encryption, and other security solutions to determine how it should be protected.
Monitor and update the classification system
Classification policies must be dynamic to accommodate the ever-changing nature of data privacy and compliance and the fact that files are created, copied, moved, and deleted every day. Establish a consistent administration process to ensure the data classification system is operating optimally and continues to meet the organization’s needs.
Data classification roles in the enterprise
Data classification is not one person’s job — it’s everyone’s job. To optimize data classification programs, organizations should designate individuals who will be responsible for carrying out specific duties. For example, Forrester defines data classification roles and responsibilities in six ways.
Data Champions
A person is responsible for the organization’s use of data for business purposes. They have an incentive to ensure that data is protected and used appropriately. This role can come in different forms, such as a Chief Privacy Office (CPO) who is responsible for data strategy, including quality, governance, and monetization. What’s important is to ensure that an identified business stakeholder will support and drive data classification efforts as a part of the organization’s overall data strategy.
Data Owners
These are the people ultimately responsible for the data and information collected and maintained by his or her department or division. They are usually a member of senior management, and can also be line-of-business managers, division heads, or the equivalent. If the data resides and is primarily in use within their group, they own it. The aim is for data owners to provide an additional layer of context for classification, such as third-party agreements, which some of today’s automated tools can’t do yet.
Data Creators
Unless an organization has an automated data classification system, the responsibility of identifying new, freshly created pieces of data (including copies of existing data) as sensitive or not rests with its creator. Anyone within an organization can be a data creator. Data creators can ask themselves one simple question to determine sensitivity: Would it be acceptable for this data to find its way into the public domain or a competitor’s hands? If not, it’s sensitive data and should be appropriately classified.
Data Users
Anyone who has access to this data is a data user. Data users must use data in a manner consistent with the purpose intended, and comply with this policy, and all policies applicable to data use. Those who have authorization to handle and use the data are in the best position to provide feedback or answer questions about the data classification tags. For example, they can answer questions such as:
-“Is the classification appropriate and based on how the data is used?”
-“Are there circumstances or situations where the data could be handled differently from what’s allowed under the current classification?”
Data Auditors
This may be a risk and compliance manager, a privacy officer, a data officer, or an equivalent role. The data auditor reviews the data owner’s assessment of the classification and determines if it’s in line with business partner, regulatory, and other corporate requirements. The data auditor also reviews feedback from data users and assesses alignment between actual or desired data use and current data-handling policies and procedures.
Data custodian
IT technicians or information security officers are responsible for maintaining and backing up the systems, databases, and servers that store the organization’s data. In addition, this role is responsible for the technical deployment of all of the rules established by data owners and for ensuring that the rules applied within systems are working.
How to optimize data classification
Organizations can begin classifying data as soon as their classification levels and criteria have been identified, and a process for applying the classification tags to the data has been established. This section overviews the creation of a data classification schema unique to each organization and best practices for optimizing data classification programs.
Create a data classification schema
Once a data classification framework has been created, businesses must then develop a classification schema with additional business criteria and an understanding of their specific types of sensitive data. But this is no easy task. Every organization is different, and there is no one-size-fits-all data protection strategy.
In the data classification schema, each category should detail the types of data to be included, the potential risks associated with compromise, and guidelines for handling the data.
Data classification categories
There are endless ways to classify data, but most organizations categorize or bucket data as variations of a four-level data classification schema — public, private, confidential, and restricted.
- Public — Information that is freely available and accessible to the public without any restrictions or adverse consequences, such as marketing material, contact information, customer service contracts, and price lists.
- Internal — Data with low security requirements, but not meant for public disclosure, such as client communications, sales playbooks, and organizational charts. Unauthorized disclosure of such information can lead to short-term embarrassment and loss of competitive advantage.
- Confidential — Sensitive data that if compromised could negatively impact operations, including harming the company, its customers, partners, or employees. Examples include vendor contracts, employee reviews and salaries, and customer information.
- Restricted — Highly sensitive corporate data that if compromised could put the organization at financial, legal, regulatory, and reputational risk. Examples include customers’ PII, PHI, and credit card information.
Data classification policy best practices
Implementing best practices ensures that organizations set themselves up for success with their data classification processes and gain the most value from them. They also want to avoid the pitfalls of data classification done wrong, which can create a lasting negative perception about this powerful data privacy process.
Some best practices for developing a robust and successful data classification policy include five steps.
- Implement automated, real-time, persistent data classification
The right automation system scan aids in streamlining the data classification process, automatically analyzing and categorizing data based on predetermined parameters.
- Make a commitment to data classification
Management support helps socialize the initiative from the top down and across the executive team. It sets the tone that classification is a priority and that everyone must participate. Even better, it establishes that the organization values its data and that appropriate data protection and handling are a part of the company culture.
- Create a culture of data privacy compliance
Educating data producers, consumers, and owners about their roles and responsibilities in protecting sensitive data and empowering them to help reduce your exposure is critical to shrinking your footprint. Many organizations conduct annual privacy and security training. However, it’s better to find ways to create an ever-present sense of privacy and security awareness among employees’ daily activities.
- Work with IT and the business from the start
By implementing a standardized and repeatable process with IT, organizations will be able to provide advice, guidance, and approval at every step of the process.
- Shrink the sensitive data footprint
Today’s ever-expanding stores of data make it increasingly difficult to protect data. Such a proliferation of sensitive information makes it extremely difficult to prevent breaches. Organizations should delete what is not needed and reduce the number of locations where the data is stored to protect people’s confidentiality. Data classification helps find redundant, extraneous, outdated, and forgotten data, so that it can be removed from the system. When an organization’s sensitive data footprint is reduced, data overall is easier to protect.