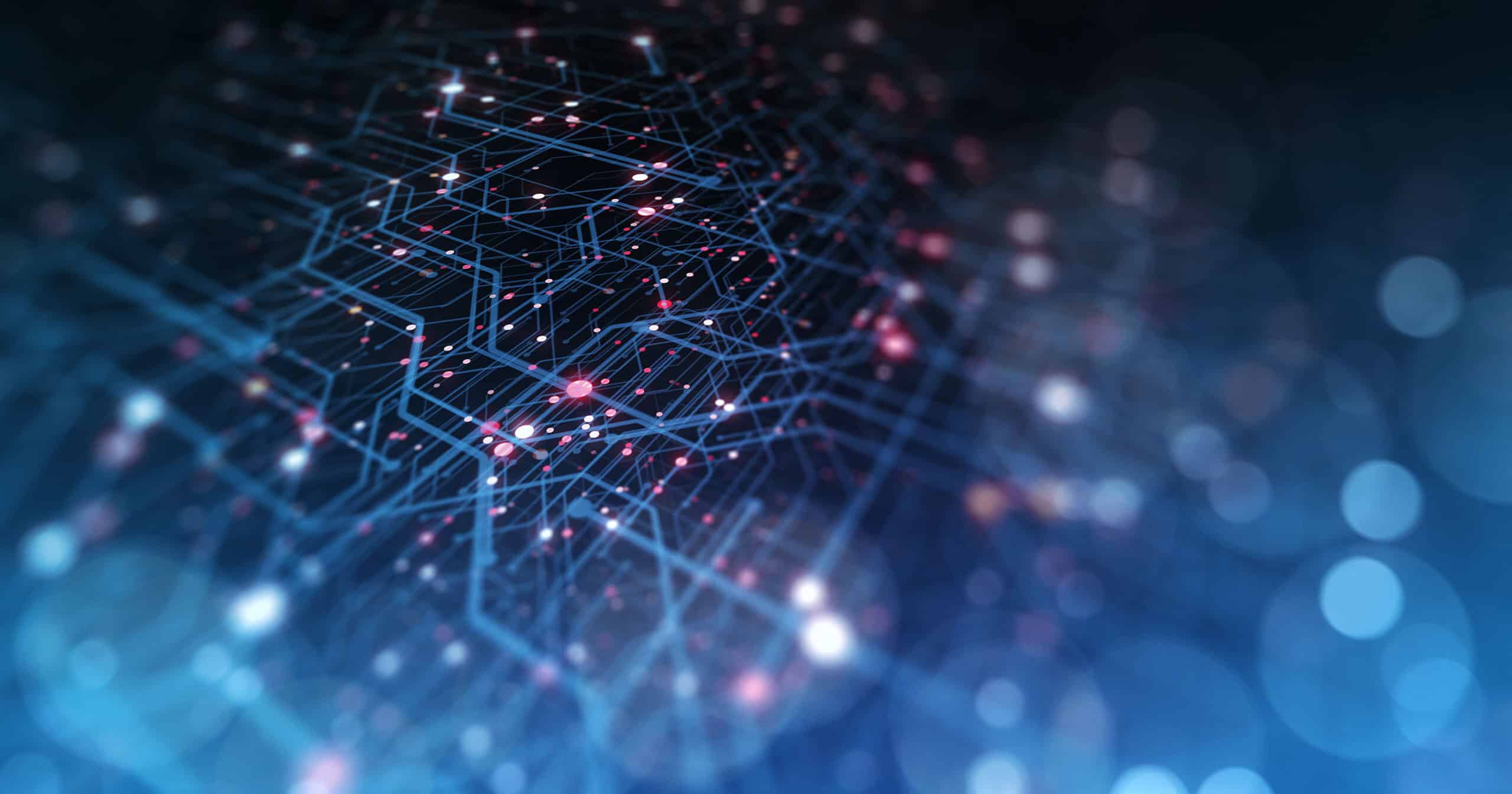
BY RYAN TULLY
October 2, 2024
It’s virtually impossible to do business today without collecting or managing sensitive data—especially if you operate within a particular industry, like finance or education, or as your business grows. Enterprises have a lot at stake when it comes to complying with data privacy laws. There are the potential risks of reputation damage, loss of customer trust and loyalty, financial losses, legal fines, and court battles.
With expanding data privacy laws, like the CCPA and CPRA, it’s essential for enterprises to not only be reactive in data privacy measures but to also be proactive. Implementing a data privacy management framework that is strategic, scalable, and agile, makes the task of safeguarding sensitive information much easier.
What are the key components of a data privacy management framework?
There are five key stages of readiness when it comes to developing a data privacy management framework. While each stage may have multiple tasks that fall underneath it, all of those tasks relate to the stage’s core behavior.
- Data discovery. Understanding what your organization is currently dealing with in terms of sensitive data is the critical first step. This means discovering what types of sensitive data you have collected and stored, where that sensitive data resides, who has access to this data and why your organization is handling the data.
When your team goes through this exercise, you may realize that your organization has unnecessarily held on to sensitive data for too long, or that you need different levels of secure storage for data of varying degrees of sensitivity. - Data classification. Classification is another foundational step to protecting sensitive data. By creating classification designations and labeling every piece of data, it becomes much easier for your team to follow protocol. For instance, you can ensure that only certain employees have access to data labeled as “sensitive” or “confidential.” By classifying data, it becomes much easier to track certain data sets when necessary, and it also makes it easier for security teams to make sense of all data at hand. Your team should be able to connect with and get a clear picture of your organization’s data landscape. Understanding everything from where it’s stored to how accessible it is, makes it easier for security leads to be proactive and protect sensitive data from potential security threats.
- Govern privacy risks. Create data privacy policies that clearly define your organization’s objectives and priorities. Your organization will also need to create a risk management strategy, along with processes and procedures that have assigned roles and responsibilities for everything from day-to-day tasks to events such as a data breach.
- Education and training. With the previous steps accomplished, you have a strong foundation to begin building awareness and educating your entire organization on data privacy best practices. Sensitive data has a way of ending up in unlikely places and can spread quickly digitally. This is one of many reasons why data privacy shouldn’t be a responsibility left solely on your IT and cybersecurity teams. Data privacy needs to be an entire team initiative, ingrained in the company culture. No matter your employees’ role, they should understand the basic requirements and expectations when working with sensitive data. This helps reduce risk and builds a strong privacy-forward mindset.
- Real-time monitoring. Real-time monitoring, alerts, and regular review of sensitive data activity are all essential to remaining proactive and complying with data privacy laws. Automation makes this endeavor easier (and less prone to human error). The ability to monitor multiple endpoints, from the cloud to on-premise systems, is also key.
What about GAPP and PMF?
The Generally Accepted Privacy Principles (GAPP) is a popular framework originally used for the accounting industry, which also happens to properly address data privacy. Later, the Privacy Management Framework (PMF) was developed as an update.
Although this framework was originally intended for the accounting industry, it is a good example of a framework with principles that touch on the three key stages of readiness we outlined above. The nine principles of PMF include:
- Management
- Agreement, notice, and communication
- Collection and creation
- Use, retention, and disposal
- Access
- Disclosure to third parties
- Security for privacy
- Data integrity and quality
- Monitoring and enforcement
As you can see, many of these principles fall under the key component areas we’ve highlighted. You may find other frameworks to model your own after, and they tend to follow the core concepts we’ve covered here in this post.
Why do enterprises need a data privacy management framework?
Adopting a data privacy management framework means never having to start from square one all over again. Frameworks are not rigid; instead, they are flexible and help guide you based on core principles. The beauty of a data privacy management framework is that even as laws and regulations change, tasks can be added on or adapted to meet evolving needs.
How building a data privacy management framework helps anticipate future regulation
With every change in privacy law, your organization can quickly adapt. When implemented early on, this framework can even allow you to be proactive. For example, organizations that have implemented a strong data privacy management framework can begin making changes now to stay ahead of the eventual needs of the CPRA, which took effect on January 1, 2023.
DPM tools that make building a framework easier
With data discovery and data classification as two foundational components of any data privacy management framework, it should be a given that DPM tools that excel in these areas are the best solution for enterprises. Without these two steps, organizations are likely to experience gaps in their privacy measures. Spirion’s Sensitive Data Platform is an all-in-one solution that leads in data discovery, automated data classification, and real-time monitoring across multiple endpoints.